Is Targeting 100% Code Coverage Bad?
TLDR; No, if supported by other tools. Yes, if that is your only target.
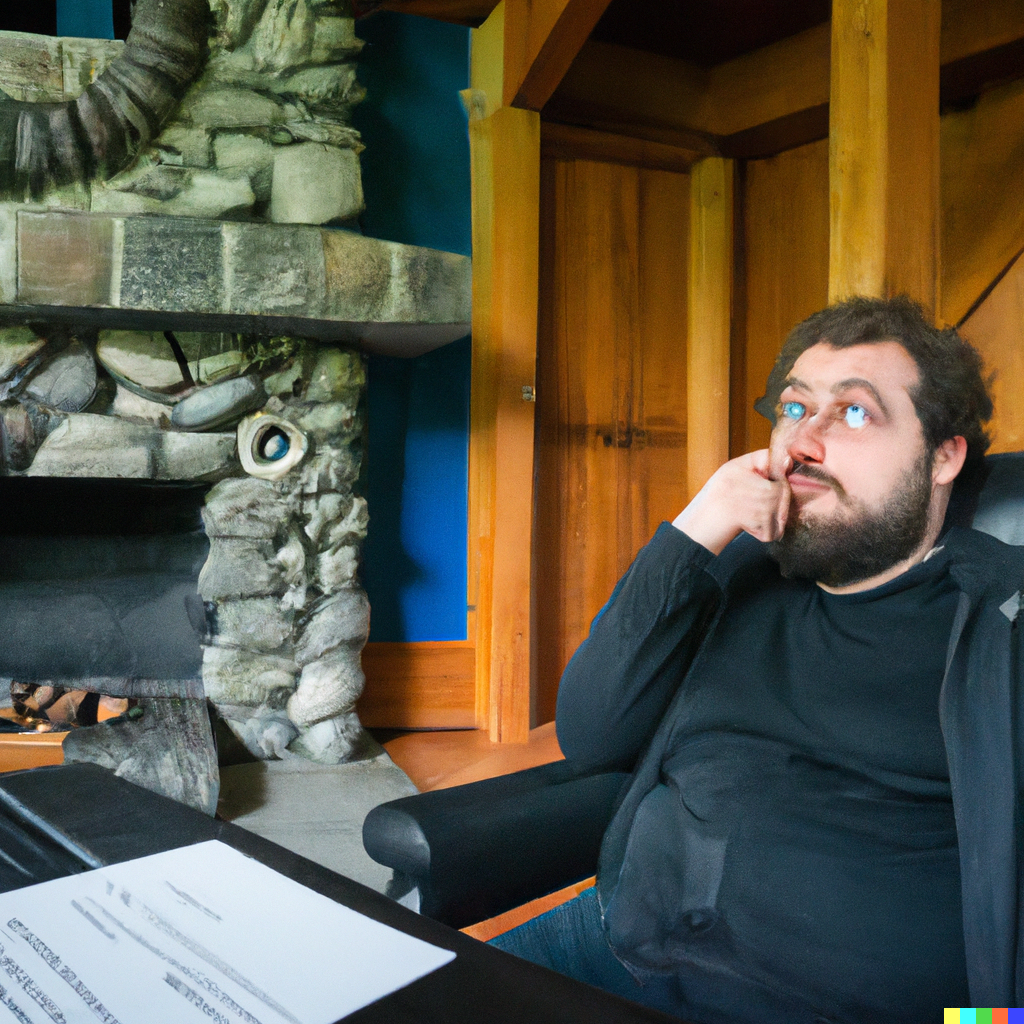
Recently I was in a discussion in my current company where a respected colleague told me that aiming for a 100% code coverage is considered bad practice.
For context, my team is developing one of the services in an event driven system. Feeling lucky that I got the chance to develop something from scratch, I set the minimum required code coverage percentage as 100 in our CI pipeline. We never went under 100 since.
My colleague told me that I could find many blog posts around the internet explaining why it is considered bad practice.
Previously, I worked on many projects where there was very little or no code coverage at all. My personal record (excluding personal projects) was 42% and even that project had zero coverage when I joined the company. I had to push and convince the management and the engineers to write tests and increase the coverage.
I know from experience how bad and how hard is to work in an environment where there are no tests at all. Any change has the possibility to break totally unrelated parts of the project and you might never find out until a customer stumbles upon that part of your product. This was so frustrating that when I got the chance to work on a new project from scratch, I set the minimum code coverage requirement to 100.
Going back to our conversation with the colleague; right after our talk I quickly searched for some blog posts explaining why aiming for 100 is considered bad. Now I will go through the process here once again and I’ll do my best to explain how this might actually be a good idea.
So I searched for “100 code coverage bad“. The search was in robot language but it did the job:
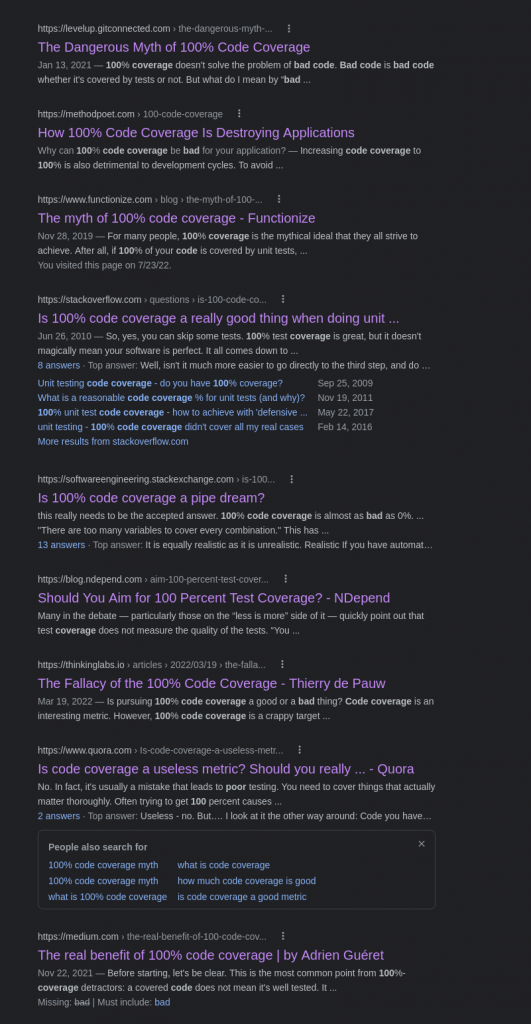
I’ve read all the links in the first page and all the answers in the stackoverflow page.
First of all, no post is directly saying 100% code coverage is bad. Most of them say things like:
“It’s bad if done poorly”,
“It’s bad if you start with zero coverage in a big project”,
“Just aiming for 100% coverage is not enough by itself”.
The last post from the screenshot is even defending 100% code coverage and I am sure I would be able to find many other examples for both sides and possibly many other valid views.
I feel like I should point out that I am well aware that blindly aiming for 100% code coverage is bad and it doesn’t guarantee anything. That’s why there are other tools you should integrate to your pipeline and/or development flow to help you understand and later increase the level of quality of your project.
Mutation Tests
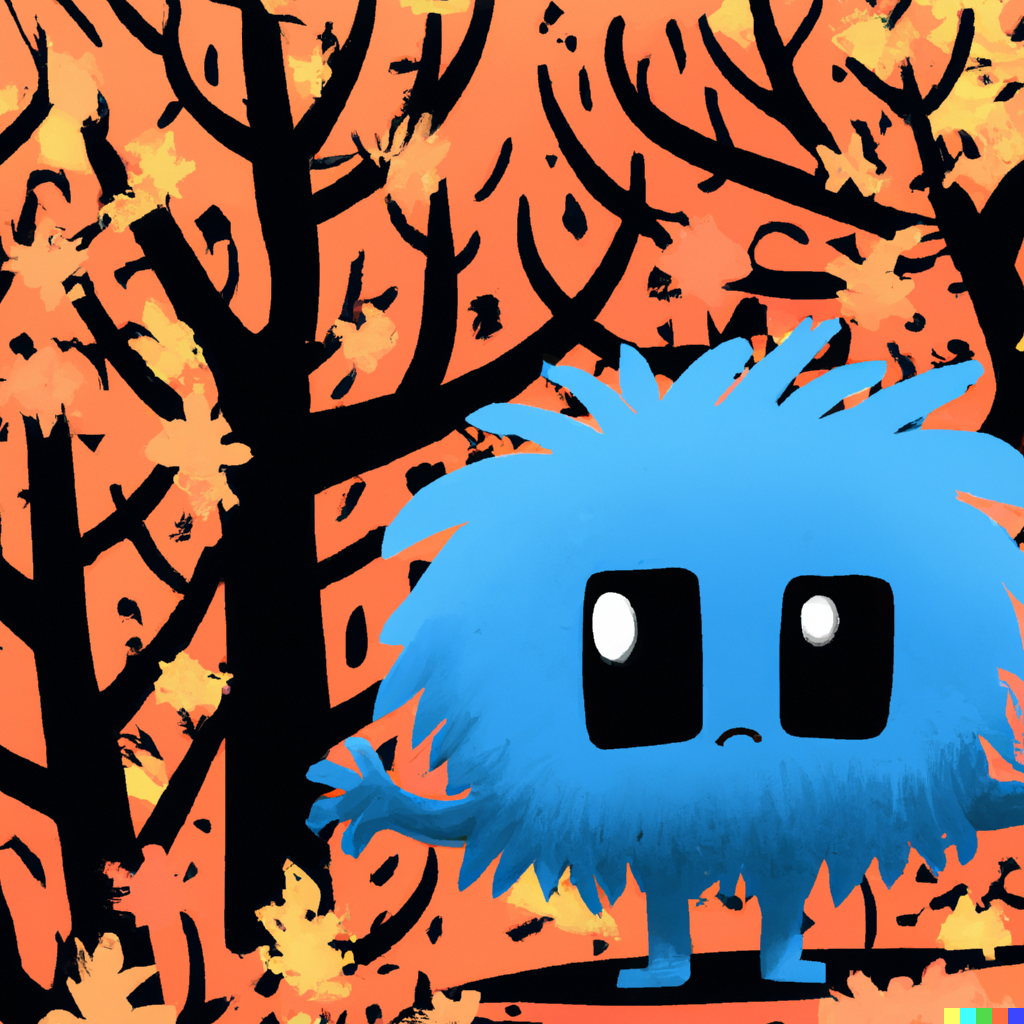
Mutation testing is a way to help you determine if your code coverage is good or bad. It measures your tests success to changes in your code so even if you have 100. For example, let’s look at the following code and pretend this is our project:
# main.py def sum(x, y): return x + y # tests.py def test_sum(): assert 0 == sum(0, 0)
In this very simple example we have a 100% code coverage. It is very obvious that the test is bad but still, I cannot say that this won’t ever happen, I can almost guarantee that somewhere in the world some engineer will write a test equivalent (in uselessness) to this one. Maybe s/he was tired that day, no blaming.
To protect ourselves against these kinds of situations we set up mutation tests. The mutation test run will analyze your code and will change things around one by one and run all your tests for each change (it will take a very long time so better to run mutations concurrently in many parallel pipelines). For this example the mutation test run will change to code to “x – y” and see if any of your tests fail. Our test_sum() function won’t fail because zero minus zero is still zero. Now, we have what we call a mutant that managed to stay alive. Our test wasn’t able to kill this mutant. One out of one alive mutants is still alive and this means our test just sucks. We are made aware by the mutation test run that we should either fix our current test or add more tests to cover this specific mutant.
# tests.py def test_zero_sum(): assert 0 == sum(0, 0) def test_sum(): assert 5 == sum(2, 3)
In the above example we just fixed the issue by adding another test. If we ran the same mutation test again our “test_sum()” would fail and kill the mutant hence giving us 100% mutation test coverage. Having 100% test coverage plus 100% mutation test coverage tells us that our code is better protected against simple changes. I am well aware that even with 100% test coverage and 100% mutation test coverage your complex logic might fail somewhere. This doesn’t mean all this coverage is useless, it just means you need another test to cover your high complexity bug. Aiming for 100 code coverage with a high percentage (above 80% maybe?) mutation test coverage will help you sleep better and be more confident when merging new code.
Code Quality
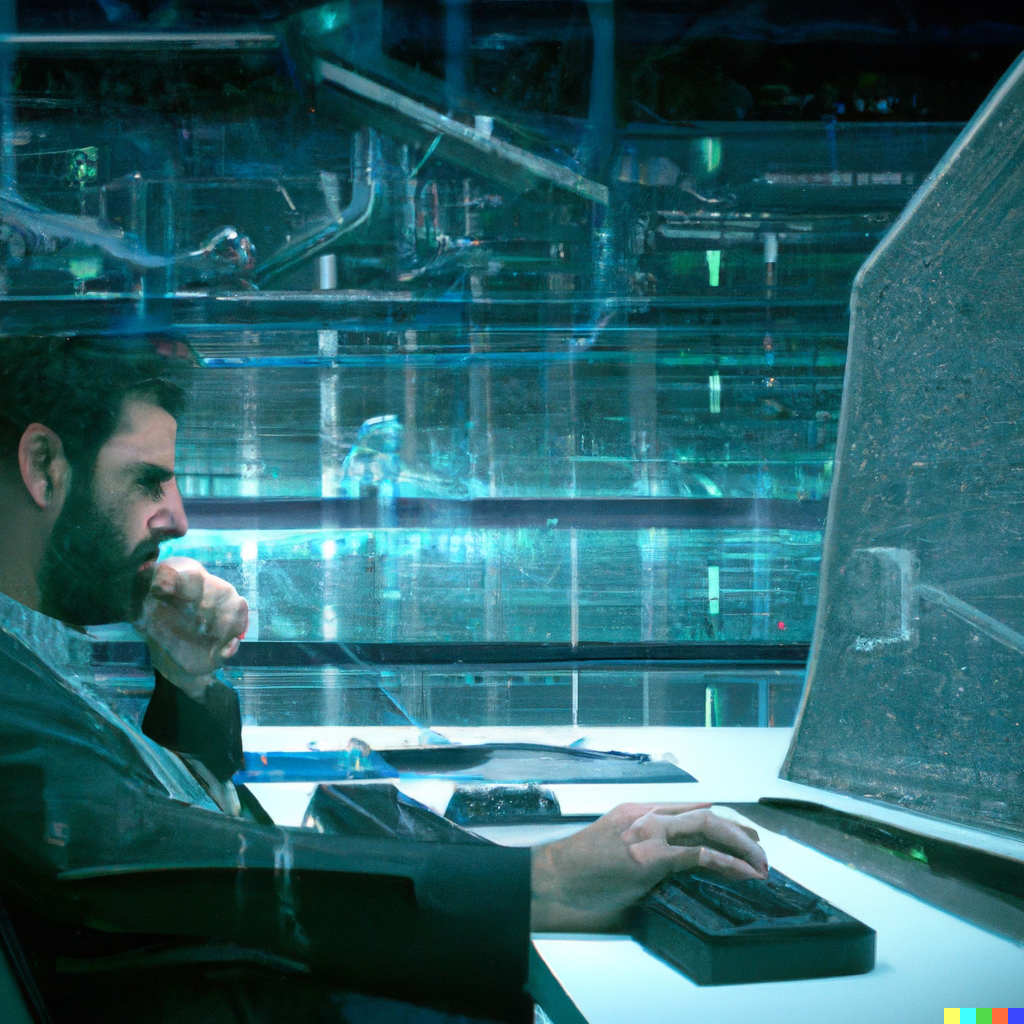
In my early days as a computer science student the only conventions that I was aware of were camel case vs snake case. If you are using Java, the convention is usually camelCase and if you are using python you use snake_case. You should be consistent in your project by sticking to either one of them so your project doesn’t burn unnecessary brain power of other developers by making them switch from one convention to the other.
Turns out there is much more to this. There are programming styles with tools that check your code style on every commit. Tools that check your import statements (depending on your programming language) and automatically order them alphabetically. Tools to check the overall code quality of your project. There are measurements to calculate your code complexity like cyclomatic complexity and/or cognitive complexity. Tools that parse your code and warn you for duplicate code, long functions and long files and so on…
These tools help you keep things clean and when things are clean every aspect of your development process will be better. You will get more readable code hence less time to understand the code. You will get smaller low complexity functions which are much easier to test.
Engineers will be happier, more motivated and highly confident because everything is easier to do in these kinds of projects.
An Example
Lets say with are working on the following tech stack:
- A Python project.
- Automated checks on every commit with git pre-commit hooks.
- Code quality checks and minimum code coverage check on Gitlab CI/CD.
- Mutations tests with mutmut.
Python Project
For our project we can use the following tools:
- Flake8 for style guide enforcement.
- Black for automated code formatting.
- isort to sort your imports automatically.
- mypy for static type checking.
Set up pre-commit Hooks
The pre-commit project will save you huge amounts of time by automatically checking things you specify at every commit. Here is an example .pre-commit-config.yaml:
repos: - repo: https://github.com/pre-commit/pre-commit-hooks rev: v3.2.0 hooks: - id: trailing-whitespace - id: end-of-file-fixer - id: check-yaml - id: debug-statements - id: check-ast - id: check-added-large-files exclude: ^static/ - repo: local hooks: - id: black name: Black description: "Black: The uncompromising Python code formatter." entry: poetry run black exclude: ^.*\b(migrations|schema_registry)\b.*$ types: [python] language: system - id: flake8 name: Flake8 description: "`flake8` is a command-line utility for enforcing style consistency across Python projects." entry: poetry run flake8 types: [python] language: system - id: isort name: isort (python) description: "isort your imports, so you don't have to." entry: poetry run isort exclude: ^.*\b(migrations|schema_registry)\b.*$ types: [python] language: system
CI Tools
I am not going into much detail here. The point is that you should set up a code quality analyzer in your CI pipeline. For example gitlab offers built in support for code climate. You can set up some configuration files in your pipeline config and you are ready to go.
The code quality tool will automatically generate reports like these:
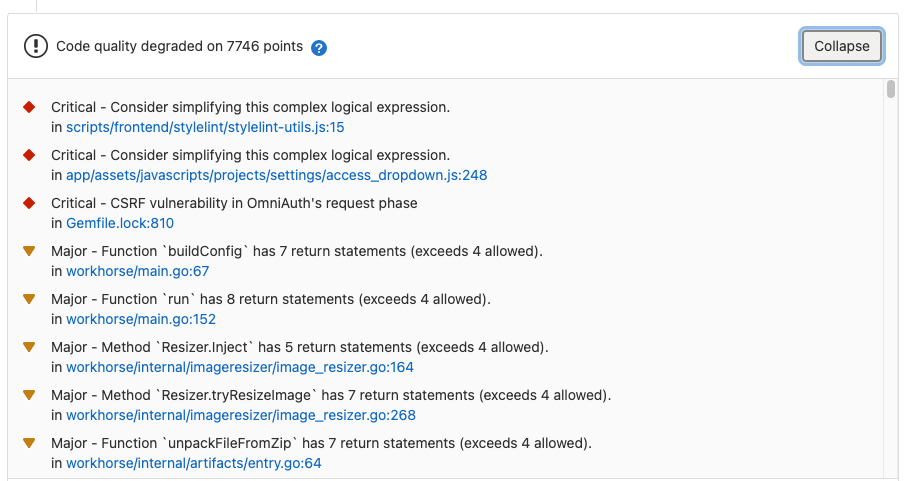
You just have to set your teams culture so that the team prioritizes code quality issues. This will help you keep your code clean and maintainable thus greatly lowering your development costs.
Mutation Tests
For our python example there is a library called mutmut. For me it did the job out of the box. I just had to add some ignores and other small optimizations for some mutations that didn’t really make sense in our case.
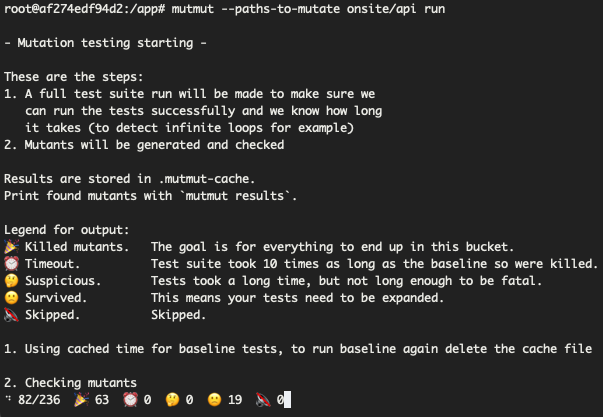
Since every single mutation has to run your entire test suite, mutation tests take a very long time to complete so it’s better if you schedule a pipeline to run concurrently on your code repository of choice. Running pipelines costs some money so to keep things cheaper we run mutation tests once a month but running once a week would be fine if you have the bandwidth to handle them.
Conclusion
Like most subjects in our field there is no definitive answer. It’s another “it depends on other things” case. 100% code coverage is good if you support it with other things such as the ones I described and possibly much more. It is also better if you start your project from scratch and aim for the hundred from the very beginning. If you join a project after years of development and there is no coverage at all then aiming for 100 doesn’t really make sense. It is probably best to try to cover as much as possible and aim for most critical parts of the system in such cases.
I see and hear a lot of “80-85% is enough” statements but I don’t think that’s the case. You see, now you have to decide what stays in the untested 20%. Why wouldn’t you test that? What if there’s a bug in that code? Do you write a test or fix the bug and leave it like that? Since there’s no obligation to satisfy a percentage any engineer can leave or forget to write tests for a critical part of a feature. Code reviews might catch some of these but ultimately the code review process is just another human being trying to find bad stuff in your code.
My conclusion (these days) is: Aiming for 100% code coverage + 100% mutation test coverage + high quality code is the way to go.
Leave a Reply